Share
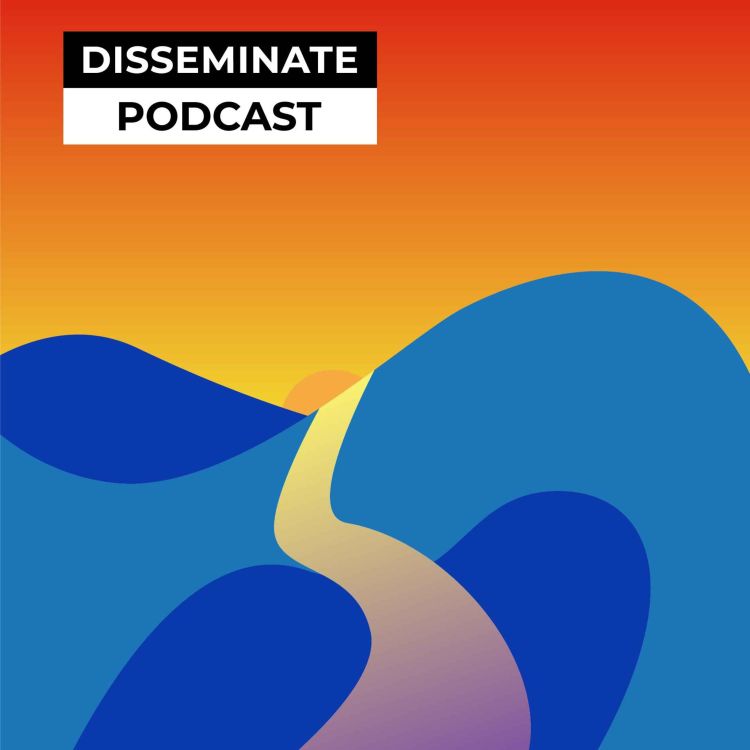
Disseminate
Harry Goldstein | Property-Based Testing | #55
Season 6, Ep. 15
•
In this episode, we chat with Harry Goldstein about Property-Based Testing (PBT). Harry shares insights from interviews with PBT users at Jane Street, highlighting PBT's strengths in testing complex code and boosting developer confidence. Harry also discusses the challenges of writing properties and generating random data, and the difficulties in assessing test effectiveness. He identifies key areas for future improvement, such as performance enhancements and better random input generation. This episode is essential for those interested in the latest developments in software testing and PBT's future.
More episodes
View all episodes
17. Matt Perron | Analytical Workload Cost and Performance Stability With Elastic Pools | #57
52:10||Season 6, Ep. 17In this episode, we dive deep into the complexities of managing analytical query workloads with our guest, Matt Perron. Matt explains how the rapid and unpredictable fluctuations in resource demands present a significant challenge for provisioning. Traditional methods often lead to either over-provisioning, resulting in excessive costs, or under-provisioning, which causes poor query latency during demand spikes. However, there's a promising solution on the horizon. Matt shares insights from recent research that showcases the viability of using cloud functions to dynamically match compute supply with workload demand without the need for prior resource provisioning. While effective for low query volumes, this approach becomes cost-prohibitive as query volumes increase, highlighting the need for a more balanced strategy.Matt introduces us to a novel strategy that combines the best of both worlds: the rapid scalability of cloud functions and the cost-effectiveness of virtual machines. This innovative approach leverages the fast but expensive cloud functions alongside slow-starting yet inexpensive virtual machines to provide elasticity without sacrificing cost efficiency. He elaborates on how their implementation, called Cackle, achieves consistent performance and cost savings across a wide range of workloads and conditions. Tune in to learn how Cackle avoids the pitfalls of traditional approaches, delivering stable query performance and minimizing costs even as demand fluctuates wildly.Links:Cackle: Analytical Workload Cost and Performance Stability With Elastic Pools [SIGMOD'24]Matt's Homepage6. High Impact in Databases with... Andreas Kipf
53:06||Season 7, Ep. 6In this High Impact episode we talk to Andreas Kipf about his work on "Learned Cardinalities". Andreas is the Professor of Data Systems at Technische Universität Nürnberg (UTN). Tune in to hear Andreas's story and learn about some of his most impactful work.The podcast is proudly sponsored by Pometry the developers behind Raphtory, the open source temporal graph analytics engine for Python and Rust.Papers mentioned on this episode:Learned Cardinalities: Estimating Correlated Joins with Deep Learning CIDR'19The Case for Learned Index Structures SIGMOD'18Adaptive Optimization of Very Large Join Queries SIGMOD'18You can find Andreas on:TwitterLinkedIn Google ScholarData Systems Lab @ UTN16. Marvin Wyrich & Justus Bogner | How Software Engineering Research Is Discussed on LinkedIn | #56
47:53||Season 6, Ep. 16In this episode, we delve into the intersection of software engineering (SE) research and professional practice with experts Marvin Wyrich and Justus Bogner. As LinkedIn stands as the largest professional network globally, it serves as a critical platform for bridging the gap between SE researchers and practitioners. Marvin and Justus explore the dynamics of how research findings are shared and discussed on LinkedIn, providing both quantitative and qualitative insights into the effectiveness of these interactions. They reveal that a significant portion of SE research posts on LinkedIn are authored by individuals outside the original research team and that a majority of comments on these posts come from industry professionals, highlighting a vibrant but underutilized avenue for science communication.Our guests shed light on the current state of this metaphorical bridge, emphasizing the potential for LinkedIn to enhance collaboration and knowledge exchange between academia and industry. Despite the promising engagement from practitioners, the discussion reveals that only half of the SE research posts receive any comments, indicating room for improvement in fostering more interactive dialogues. Marvin and Justus offer practical advice for researchers to better engage with practitioners on LinkedIn and suggest strategies for making research dissemination more impactful. This episode provides valuable insights for anyone interested in leveraging social media for advancing software engineering knowledge and practice.Links:ICSE'24 PaperMarvin's HomepageJustus's Homepage5. High Impact in Databases with... Joe Hellerstein
52:56||Season 7, Ep. 5In this High Impact episode we talk to Joe Hellerstein.Joe is the Jim Gray Professor of Computer Science at UC Berkeley. Tune in to hear Joe's story and learn about some of his most impactful work.The podcast is proudly sponsored by Pometry the developers behind Raphtory, the open source temporal graph analytics engine for Python and Rust.4. High Impact in Databases with... Raghu Ramakrishnan
23:56||Season 7, Ep. 4In this High Impact episode we talk to Raghu Ramakrishnan.Raghu is CTO for Data and a Technical Fellow at Microsoft. Tune in to hear Raghu's story and learn about some of his most impactful work.The podcast is proudly sponsored by Pometry the developers behind Raphtory, the open source temporal graph analytics engine for Python and Rust.14. Gina Yuan | In-Network Assistance With Sidekick Protocols | #54
55:25||Season 6, Ep. 14Join us as we chat with Gina Yuan about her pioneering work on sidekick protocols, designed to enhance the performance of encrypted transport protocols like QUIC and WebRTC. These protocols ensure privacy but limit in-network innovations. Gina explains how sidekick protocols allow intermediaries to assist endpoints without compromising encryption.Discover how Gina tackles the challenge of referencing opaque packets with her innovative quACK tool and learn about the real-world benefits, including improved Wi-Fi retransmissions, energy-saving proxy acknowledgments, and the PACUBIC congestion-control mechanism. This episode offers a glimpse into the future of network performance and security.Links:NSDI'2024 PaperGina's HomepageSidekick's Github Repo3. High Impact in Databases with... Moshe Vardi
47:39||Season 7, Ep. 3Welcome to another episode of the High Impact series - today we talk with Moshe Vardi! Moshe is the Karen George Distinguished Service Professor in Computational Engineering at Rice University where his research focuses on automated reasoning. Tune in to hear Moshe's story and learn about some of his most impactful work.The podcast is proudly sponsored by Pometry the developers behind Raphtory, the open source temporal graph analytics engine for Python and Rust.You can find Moshe on X, LinkedIn, and Mastadon @vardi. Links to all his work can be found on his website here.13. Tammy Sukprasert | Move Your Workloads To Sweden! | #53
32:50||Season 6, Ep. 13In this episode, we dip our toes into the world of sustainable computing and interview Tammy Sukprasert about her research on reducing carbon emissions in cloud computing through workload scheduling. Tammy explores the concept of shifting cloud workloads across different times and locations to coincide with low-carbon energy availability. Unlike previous studies that focused on specific regions or workloads, her comprehensive analysis uses carbon intensity data from 123 regions to assess both batch and interactive workloads. She considers various factors such as job duration, deadlines, and service level objectives (SLOs). Tammy's findings reveal that while spatiotemporal workload shifting can reduce carbon emissions, the practical upper bounds of these reductions are limited and far from ideal. Simple scheduling policies often achieve most of the potential reductions, with more complex techniques offering minimal additional benefits.Additionally, Tammy's research highlights that as the energy grid becomes greener, the benefits of carbon-aware scheduling over carbon-agnostic approaches decrease. This discussion offers crucial insights for the future of cloud computing and sustainable technology. Whether you're a tech enthusiast, environmental advocate, or cloud industry professional, Tammy's work provides valuable perspectives on the intersection of technology and sustainability. Join us to learn more about how innovative scheduling strategies can contribute to a greener cloud computing landscape.Links:Tammy's LinkedInOn the Limitations of Carbon-Aware Temporal and Spatial Workload Shifting in the Cloud EuroSys'24 Paper Carbon Savings Upper Bound Analysis