Share
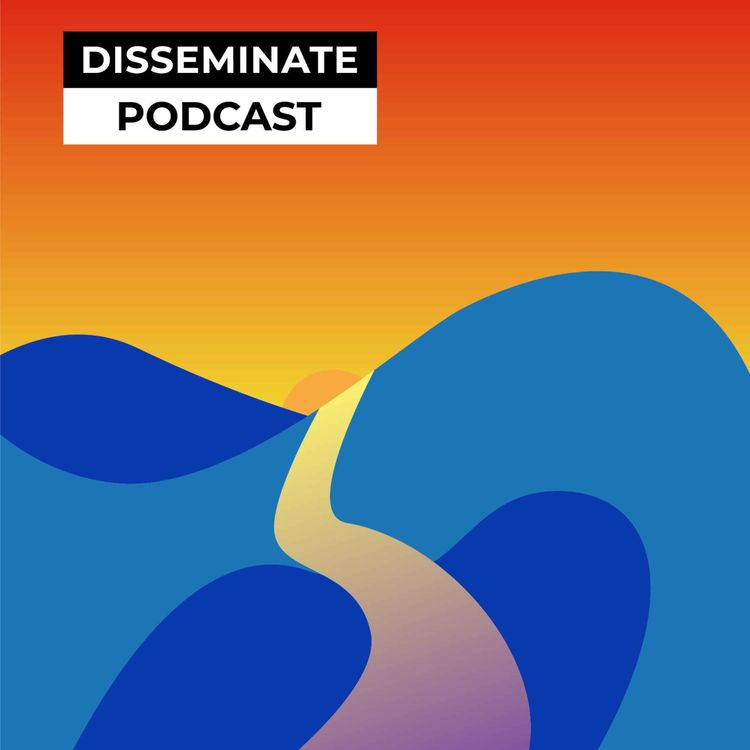
Disseminate
Matt Perron | Analytical Workload Cost and Performance Stability With Elastic Pools | #57
In this episode, we dive deep into the complexities of managing analytical query workloads with our guest, Matt Perron. Matt explains how the rapid and unpredictable fluctuations in resource demands present a significant challenge for provisioning. Traditional methods often lead to either over-provisioning, resulting in excessive costs, or under-provisioning, which causes poor query latency during demand spikes. However, there's a promising solution on the horizon. Matt shares insights from recent research that showcases the viability of using cloud functions to dynamically match compute supply with workload demand without the need for prior resource provisioning. While effective for low query volumes, this approach becomes cost-prohibitive as query volumes increase, highlighting the need for a more balanced strategy.
Matt introduces us to a novel strategy that combines the best of both worlds: the rapid scalability of cloud functions and the cost-effectiveness of virtual machines. This innovative approach leverages the fast but expensive cloud functions alongside slow-starting yet inexpensive virtual machines to provide elasticity without sacrificing cost efficiency. He elaborates on how their implementation, called Cackle, achieves consistent performance and cost savings across a wide range of workloads and conditions. Tune in to learn how Cackle avoids the pitfalls of traditional approaches, delivering stable query performance and minimizing costs even as demand fluctuates wildly.
Links:
- Cackle: Analytical Workload Cost and Performance Stability With Elastic Pools [SIGMOD'24]
- Matt's Homepage
More episodes
View all episodes
19. Raunak Shah | R2D2: Reducing Redundancy and Duplication in Data Lakes | #59
31:09||Season 6, Ep. 19In this episode, Raunak Shah joins us to discuss the critical issue of data redundancy in enterprise data lakes, which can lead to soaring storage and maintenance costs. Raunak highlights how large-scale data environments, ranging from terabytes to petabytes, often contain duplicate and redundant datasets that are difficult to manage. He introduces the concept of "dataset containment" and explains its significance in identifying and reducing redundancy at the table level in these massive data lakes—an area where there has been little prior work.Raunak then dives into the details of R2D2, a novel three-step hierarchical pipeline designed to efficiently tackle dataset containment. By utilizing schema containment graphs, statistical min-max pruning, and content-level pruning, R2D2 progressively reduces the search space to pinpoint redundant data. Raunak also discusses how the system, implemented on platforms like Azure Databricks and AWS, offers significant improvements over existing methods, processing TB-scale data lakes in just a few hours with high accuracy. He concludes with a discussion on how R2D2 optimally balances storage savings and performance by identifying datasets that can be deleted and reconstructed on demand, providing valuable insights for enterprises aiming to streamline their data management strategies.Materials:SIGMOD'24 Paper - R2D2: Reducing Redundancy and Duplication in Data LakesICDE'24 - Towards Optimizing Storage Costs in the Cloud8. High Impact in Databases with... Aditya Parameswaran
58:57||Season 7, Ep. 8In this High Impact episode we talk to Aditya Parameswaran about his some of his most impactful work.Aditya is an Associate Professor at the University of California, Berkeley. Tune in to hear Aditya's story! The podcast is proudly sponsored by Pometry the developers behind Raphtory, the open source temporal graph analytics engine for Python and Rust.Links:EPIC Data LabAnswering Queries using Humans, Algorithms and Databases (CIDR'11)Potter’s Wheel: An Interactive Data Cleaning System (VLDB'01)Online Aggregation (SIGMOD'97)Polaris: A System for Query, Analysis and Visualization of Multi-dimensional Relational Databases (INFOVIS'00)Coping with Rejection PonderYou can find Aditya on:TwitterLinkedInGoogle Scholar18. Marco Costa | Taming Adversarial Queries with Optimal Range Filters | #58
37:07||Season 6, Ep. 18In this episode, we sit down with Marco Costa to discuss the fascinating world of range filters, focusing on how they help optimize queries in databases by determining whether a range intersects with a given set of keys. Marco explains how traditional range filters, like Bloom filters, often result in high false positives and slow query times, especially when dealing with adversarial inputs where queries are correlated with the keys. He walks us through the limitations of existing heuristic-based solutions and the common challenges they face in maintaining accuracy and speed under such conditions.The highlight of our conversation is Grafite, a novel range filter introduced by Marco and his team. Unlike previous approaches, Grafite comes with clear theoretical guarantees and offers robust performance across various datasets, query sizes, and workloads. Marco dives into the technicalities, explaining how Grafite delivers faster query times and maintains predictable false positive rates, making it the most reliable range filter in scenarios where queries are correlated with keys. Additionally, he introduces a simple heuristic filter that excels in uncorrelated queries, pushing the boundaries of current solutions in the field.SIGMOD' 24 Paper - Grafite: Taming Adversarial Queries with Optimal Range Filters7. High Impact in Databases with... Ali Dasdan
01:03:02||Season 7, Ep. 7In this High Impact episode we talk to Ali Dasdan, CTO at Zoominfo. Tune in to hear Ali's story and learn about some of his most impactful work such as his work on "Map-Reduce-Merge".The podcast is proudly sponsored by Pometry the developers behind Raphtory, the open source temporal graph analytics engine for Python and Rust.Materials mentioned on this episode:Map-Reduce-Merge: Simplified Relational Data Processing on Large Clusters (SIGMOD'07)The Art of Doing Science and Engineering: Learning to Learn, Richard HammingHow to Solve It, George PolyaSystems Architecting: Creating & Building Complex Systems, Eberhardt RechtinYou can find Ali on:TwitterLinkedIn6. High Impact in Databases with... Andreas Kipf
53:06||Season 7, Ep. 6In this High Impact episode we talk to Andreas Kipf about his work on "Learned Cardinalities". Andreas is the Professor of Data Systems at Technische Universität Nürnberg (UTN). Tune in to hear Andreas's story and learn about some of his most impactful work.The podcast is proudly sponsored by Pometry the developers behind Raphtory, the open source temporal graph analytics engine for Python and Rust.Papers mentioned on this episode:Learned Cardinalities: Estimating Correlated Joins with Deep Learning CIDR'19The Case for Learned Index Structures SIGMOD'18Adaptive Optimization of Very Large Join Queries SIGMOD'18You can find Andreas on:TwitterLinkedIn Google ScholarData Systems Lab @ UTN16. Marvin Wyrich & Justus Bogner | How Software Engineering Research Is Discussed on LinkedIn | #56
47:53||Season 6, Ep. 16In this episode, we delve into the intersection of software engineering (SE) research and professional practice with experts Marvin Wyrich and Justus Bogner. As LinkedIn stands as the largest professional network globally, it serves as a critical platform for bridging the gap between SE researchers and practitioners. Marvin and Justus explore the dynamics of how research findings are shared and discussed on LinkedIn, providing both quantitative and qualitative insights into the effectiveness of these interactions. They reveal that a significant portion of SE research posts on LinkedIn are authored by individuals outside the original research team and that a majority of comments on these posts come from industry professionals, highlighting a vibrant but underutilized avenue for science communication.Our guests shed light on the current state of this metaphorical bridge, emphasizing the potential for LinkedIn to enhance collaboration and knowledge exchange between academia and industry. Despite the promising engagement from practitioners, the discussion reveals that only half of the SE research posts receive any comments, indicating room for improvement in fostering more interactive dialogues. Marvin and Justus offer practical advice for researchers to better engage with practitioners on LinkedIn and suggest strategies for making research dissemination more impactful. This episode provides valuable insights for anyone interested in leveraging social media for advancing software engineering knowledge and practice.Links:ICSE'24 PaperMarvin's HomepageJustus's Homepage5. High Impact in Databases with... Joe Hellerstein
52:56||Season 7, Ep. 5In this High Impact episode we talk to Joe Hellerstein.Joe is the Jim Gray Professor of Computer Science at UC Berkeley. Tune in to hear Joe's story and learn about some of his most impactful work.The podcast is proudly sponsored by Pometry the developers behind Raphtory, the open source temporal graph analytics engine for Python and Rust.15. Harry Goldstein | Property-Based Testing | #55
49:13||Season 6, Ep. 15In this episode, we chat with Harry Goldstein about Property-Based Testing (PBT). Harry shares insights from interviews with PBT users at Jane Street, highlighting PBT's strengths in testing complex code and boosting developer confidence. Harry also discusses the challenges of writing properties and generating random data, and the difficulties in assessing test effectiveness. He identifies key areas for future improvement, such as performance enhancements and better random input generation. This episode is essential for those interested in the latest developments in software testing and PBT's future.Links:ICSE'24 Paper Harry's websiteX: @hgoldstein95